Bayesian computation for logistic regression
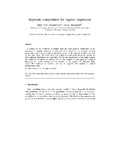
View/ Open
Date
2005Author
Mokgatlhe, L.
Groenewald, P.C.N.
Publisher
Elsevier, http://www.elsevier.comType
Published ArticleMetadata
Show full item recordAbstract
A method for the simulation of samples from the exact posterior distributions of the parameters in logistic regression is proposed. It is based on the principle of data augmentation and a latent variable is introduced, similar to the approach of Albert and chib (J. Am. Stat. Assoc. 88 (1993) 669), who applied it to the probit model. In general, the full conditional distributions are intractable, but with the introductions of the latent variable all conditional distributions are uniform, and the Gibbs sampler is easily applicable. Marginal likelihoods for model selection can be obtained at the expense of additional Gibbs cycles. The technique is extended and can be applied with nominal or ordinal polychotomous data.